For precision processing companies involved in automobile manufacturing, medical device manufacturing, and other industries.
We solve industry-specific challenges in visual inspection.
Are you having problems with this?
We can solve your problems of visual inspection.
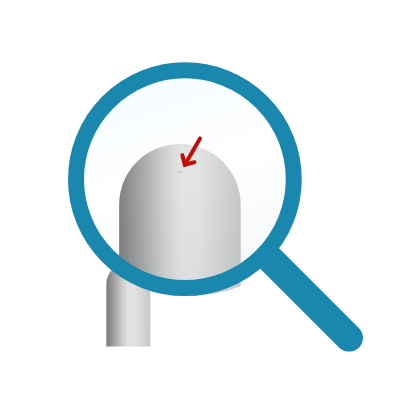
Can’t find the deficiency well.
Examples of not found
- Defects on curved or mirrored surfaces reflect light and cannot be found well
- I can’t successfully find defects that are assimilated with machining marks.
- Defects in transparent bodies are not found well because light is deflected and reflected.
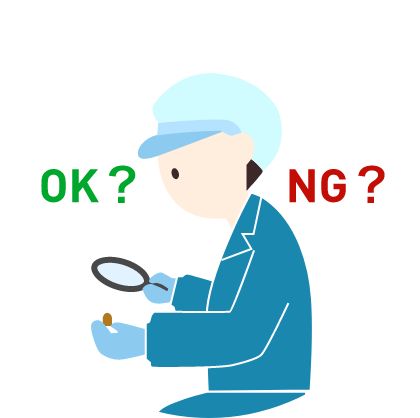
Judgment is not stable
Examples of unstable
- Dependent on humans and therefore unstable due to human error and variation
- Skilled workers are needed, but the time and cost to train them is not stable.
- Automation has been introduced, but the condition setting is difficult and judgment is not stable.
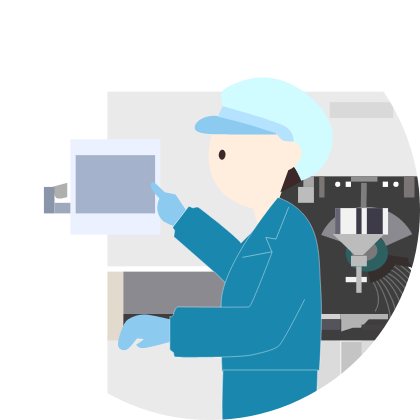
Inspection results cannot be feedback to the manufacturing process
Examples where feedback cannot be given to the manufacturing process
- Unable to quantify and analyze deficiencies.
- Inspection results cannot be reflected on the manufacturing floor as quickly as possible./li>
- Accumulated inspection data cannot be easily checked and utilized.
What HUMO’s automated visual inspection equipment can do
Find

Judgment

Digitizing

Manpower saving
Technology to find deficiencies
Three “image inspection technologies” make it possible to inspect curved surfaces, glossy surfaces, machining marks, transparent objects, and other difficult-to-inspect objects. Even minute defects are not missed.
1. Defects on curved and mirrored surfaces
Object: Curved surfaces with high gloss and strong halation, small parts with spherical surfaces, etc. (up to about 10 cm)
It is difficult to find defects in parts composed of curved mirror surfaces because light reflects off them, and even the human eye must observe them repeatedly from different angles to find them. It is also difficult to distinguish a defect from a shadow reflected on the surface. We have developed a method to detect defects by reflecting a specific light pattern onto the object to be inspected and analyzing the image for deformation. This method enables stable detection of defects as shallow as several tens of micrometers without the need for repeated observation from different angles.
Case study of defect detection on a curved surface
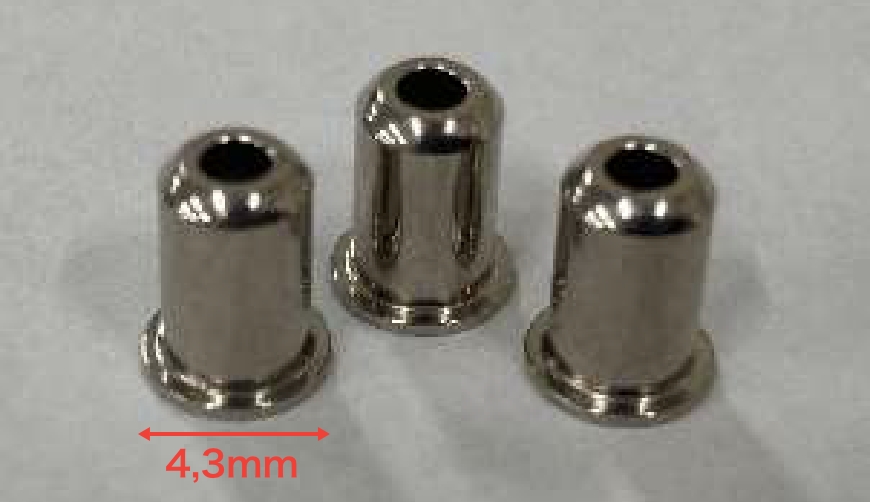
Object example: Curved surface parts
Object viewed from directly above a curved surface part
Detects microscopic scratches by irradiating patterns.
2. Defects that are assimilated with processing marks
Object: Small parts, etc., precision-machined in units of several tens of microns. (10 cm or less)
For example, it is not easy to find defects of several tens of micrometers on machined parts that have machining marks on the surface, because they are assimilated with the machining marks. We are able to distinguish between the machining marks and defects by frequency analysis of the image, and can accurately detect pits as small as a few dozen micrometers. Not only pits, but also other defects can be detected.
Case study of defect detection of machining marks
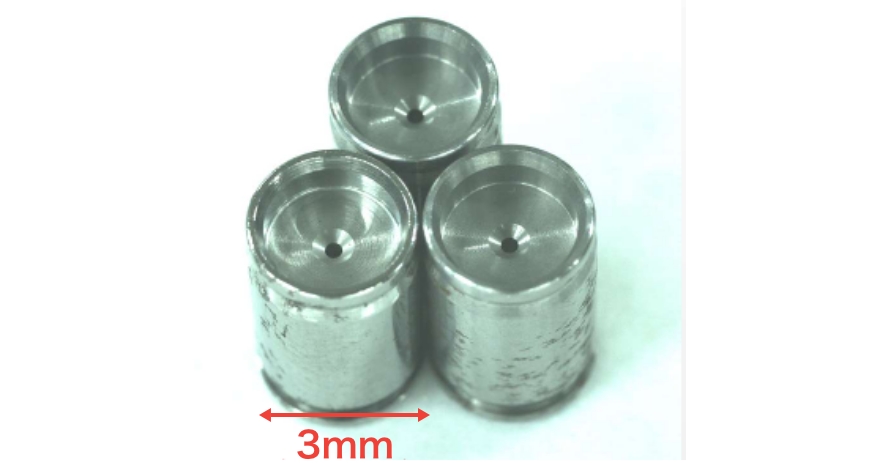
Object: Cutting parts
Cutting parts are photographed from above.
Special image processing is used to detect holes, which are difficult to distinguish from machining marks, and to detect defects.
3. Defects in transparent or translucent bodies
Object: Transparent and translucent objects
It is difficult to find defects in transparent and translucent objects because light is deflected and reflected, and conventionally these objects have been inspected by the human eye. We use multi-colored light sources and an algorithm that suppresses the effects of ambient light and individual differences in the inspected object to accurately detect the surface and internal conditions of transparent and semi-transparent objects. Any size of object can be inspected. Any material that allows light to pass through can be inspected, regardless of its hardness.
Case study of transparent body defect detection
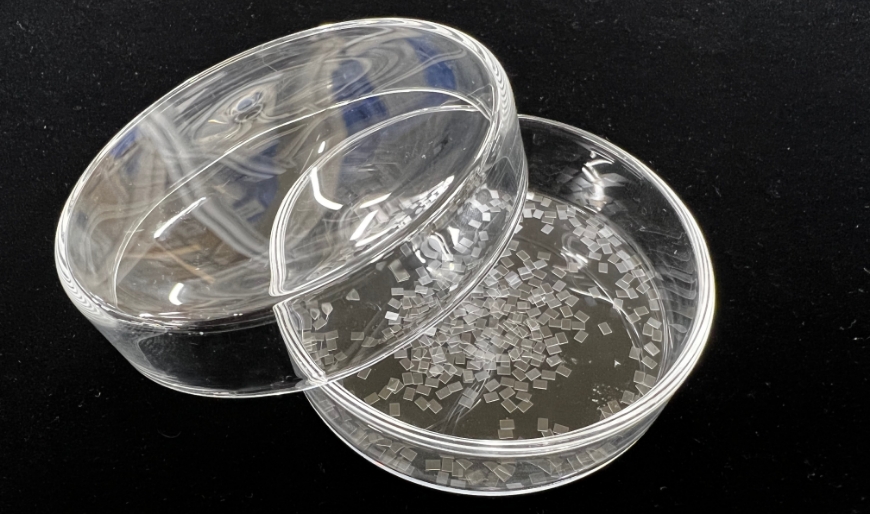
Object: Transparent (slightly milky) quartz plate
Imaging with two-color illumination
Right image: Discoloration is detected by processing only red information
Technology to determine deficiencies Technology to achieve stable determination
Obtain stable judgments through mechanization
Visual inspections by humans are prone to human error and inconsistencies in inspectors’ judgments. In contrast, automatic visual inspection, which uses image processing of camera images to make judgments, can always make stable judgments.
Stable judgments are obtained through flexible judgment algorithms and shared judgment conditions.
Although human intuitive judgment is superior to that of machines, it is difficult to accurately convey the judgment criteria to others. We use algorithms that optimize judgment criteria such as size and length of defects as well as shape characteristics and color according to the object to enable judgment that is close to human judgment, and then convert the judgment conditions into data for shared use. This enables stable judgment even when switching inspection targets or increasing the number of inspections. Translated with www.DeepL.com/Translator (free version)
Stable judgment is obtained by setting easy judgment conditions.
Even with automation, it is sometimes difficult to set the conditions for judgment, resulting in excessive judgment, which worsens the yield rate, or conversely, NG judgment may be too lenient. We combine intermediate judgments based on machine learning with judgments based on numerical conditions to make realistic judgments that are not excessive.
Machine learning usually requires the registration of about 10,000 samples, and the registration process is a challenge.
By limiting the use of machine learning to intermediate judgments, we are able to make stable judgments with only about 100 registered good samples.
Technology to feed back inspection results to the manufacturing process
Deficiencies can be quantified and classified.
Visual inspection is an essential process, but it cannot improve quality. To improve quality, it is necessary to collect data on defects and provide feedback to the manufacturing process. By systematizing visual inspection, defects can be quantified, classified, and totaled to accumulate and utilize the data.
Quickly reflect inspection results.
Our equipment not only classifies and stores individual defect data, judgment results, classification statistics, and image data, but also statistically processes judgment results and provides quick feedback to the manufacturing process through a function that allows users to check the inspection status in real time.
Accumulated data can be easily utilized.
We provide real-time data sharing and aggregation functions using a network, and offer a centralized management system to meet your needs. This makes it easy to consolidate and utilize data even for multiple devices and a huge number of inspections.
About Humo Laboratory
Efforts for manpower-saving technology and automatic inspection equipment
Inspections that rely on human senses require securing inspectors and training their skills. Furthermore, it is impossible to reduce human error and variation to zero. The best way to solve these problems is to save manpower by introducing automatic appearance equipment.
In addition, yield improvement and quality control have become extremely important issues in today’s manufacturing sites, and a system that can be linked to the production line is essential for inspection equipment.
In addition to technologies for detecting and determining defects, we have conveyance, control, and network technologies accumulated through 50 years of experience with automatic inspection equipment for electronic components. By combining these technologies, we propose automatic visual inspection equipment with an easy-to-use man-machine interface that systematizes the process from supply, transport, inspection, classification, data processing, and consolidation.
Translated with www.DeepL.com/Translator (free version)
According to one survey, the manpower saving in the visual inspection process is less than 40%, including semi-automatic inspection. There are various reasons for not being able to reduce manpower, and we can help you solve them.
Main client companies
TAIYO YUDEN CO.,LTD., KYOCERA Corporation, Murata Manufacturing Co., Ltd., YAGEO, Samsung Electro-Mechanics, CCTC, Daishinku, CITIZEN FINEDEVICE CO.,LTD., Nihon Denpa Kogyo and many more
please
don’t hesitate to contact
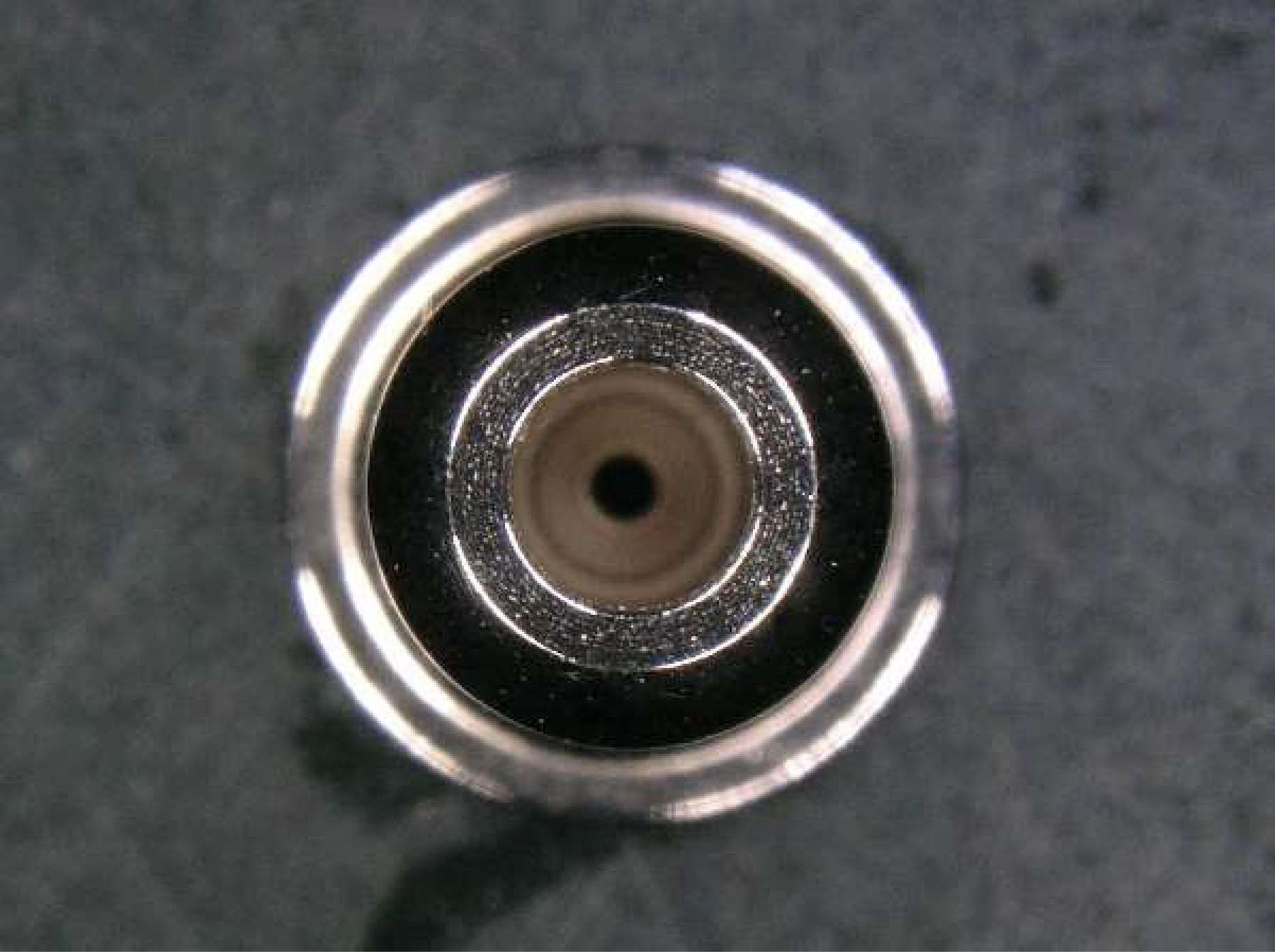
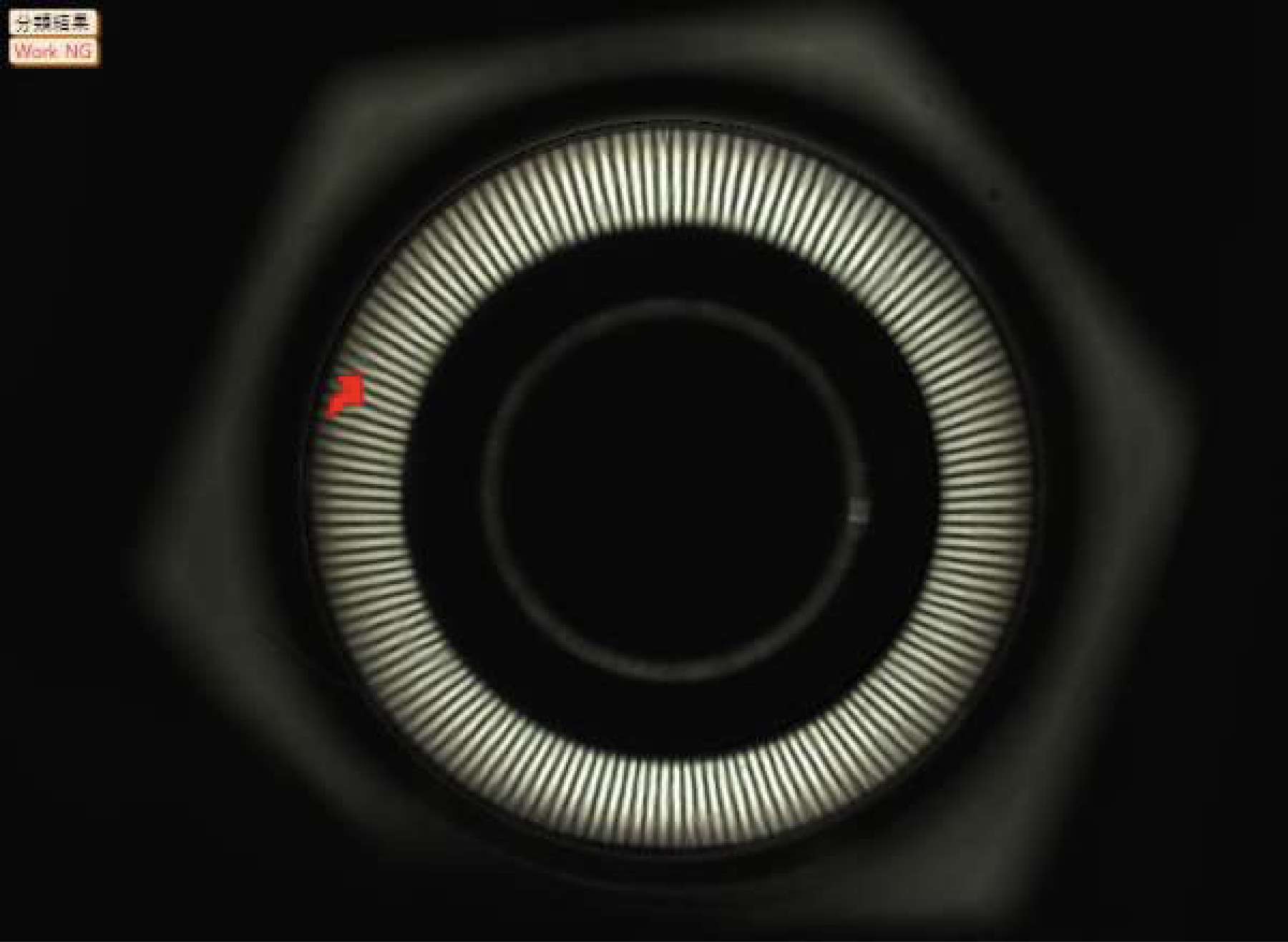
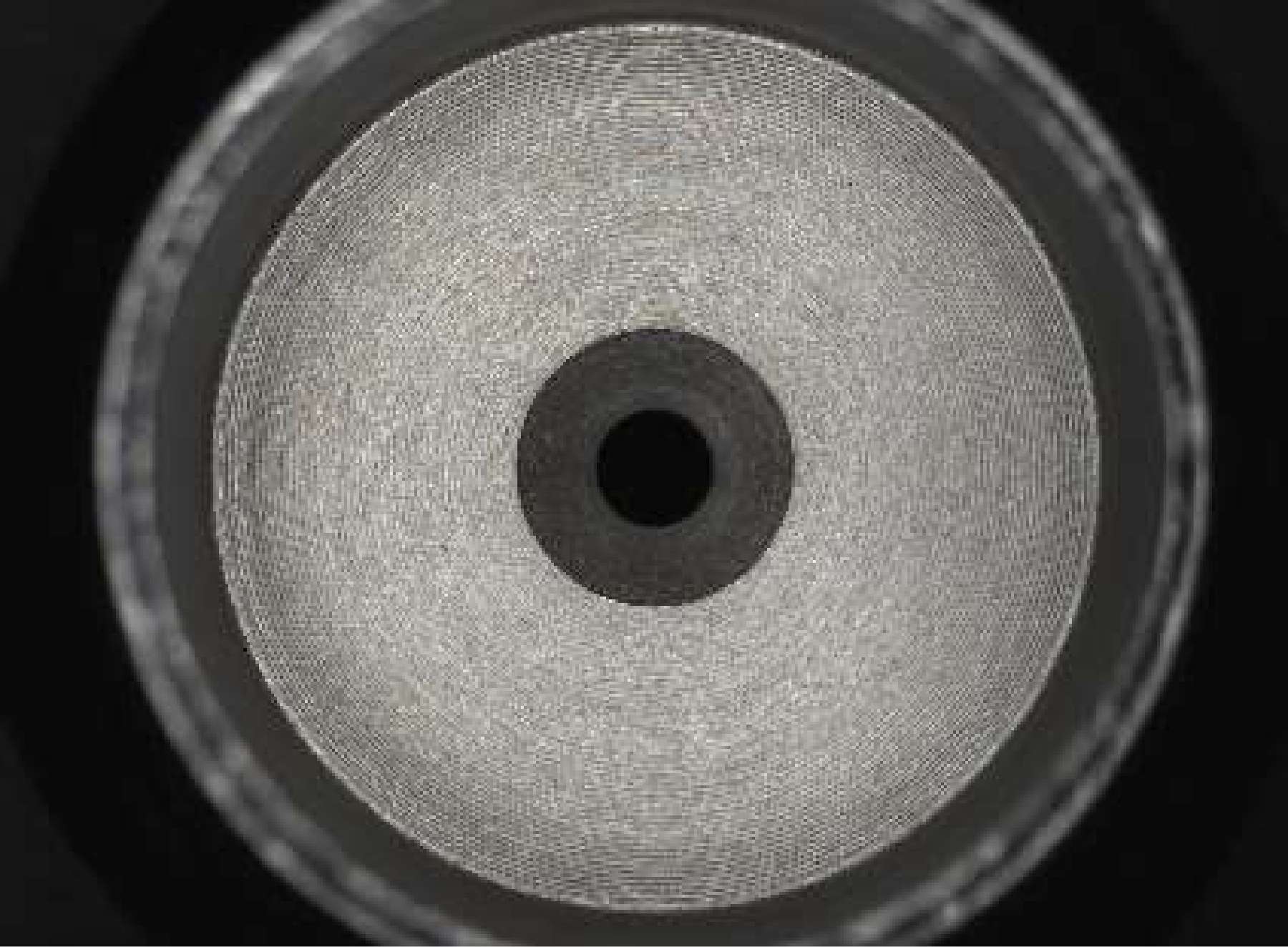
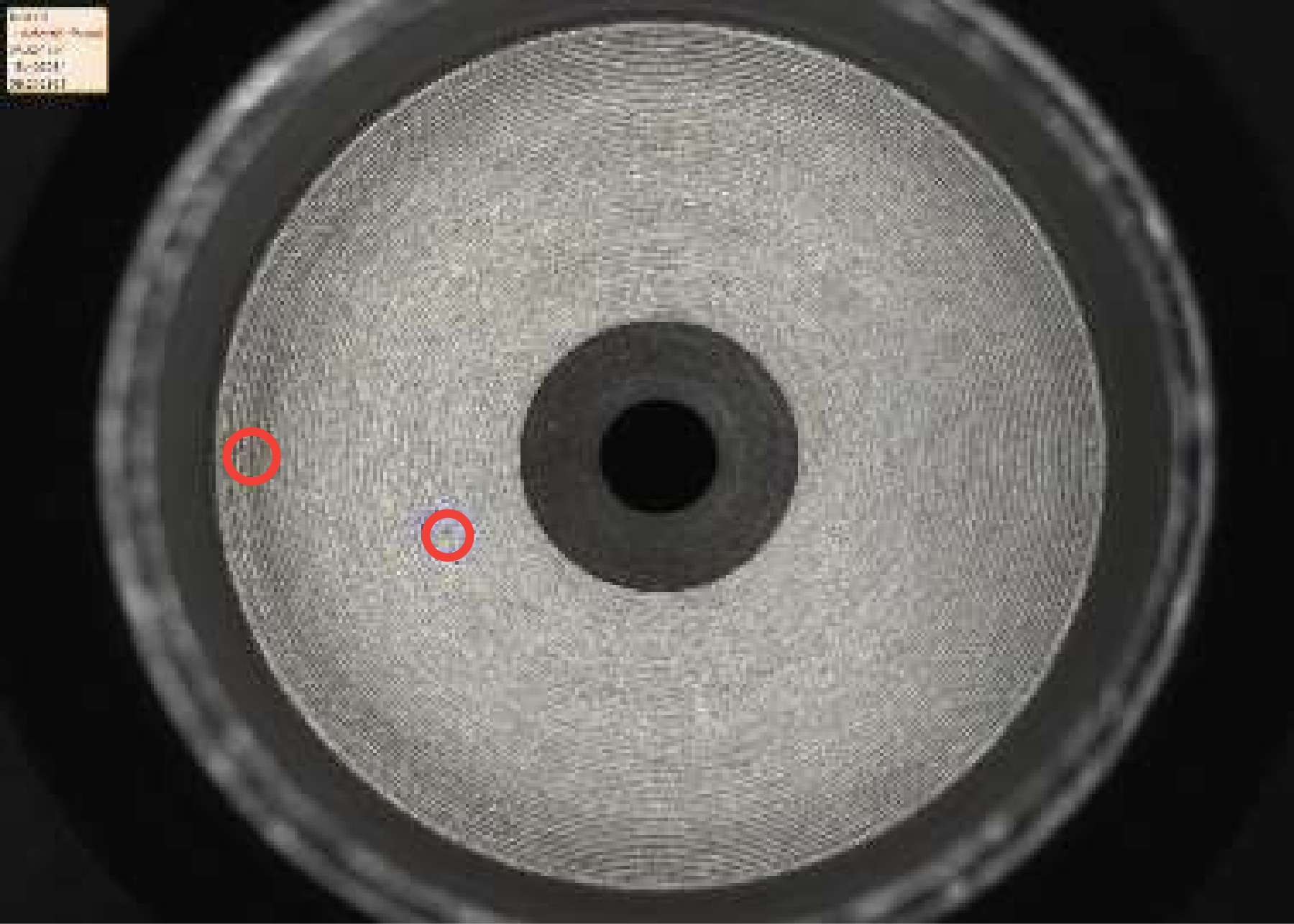
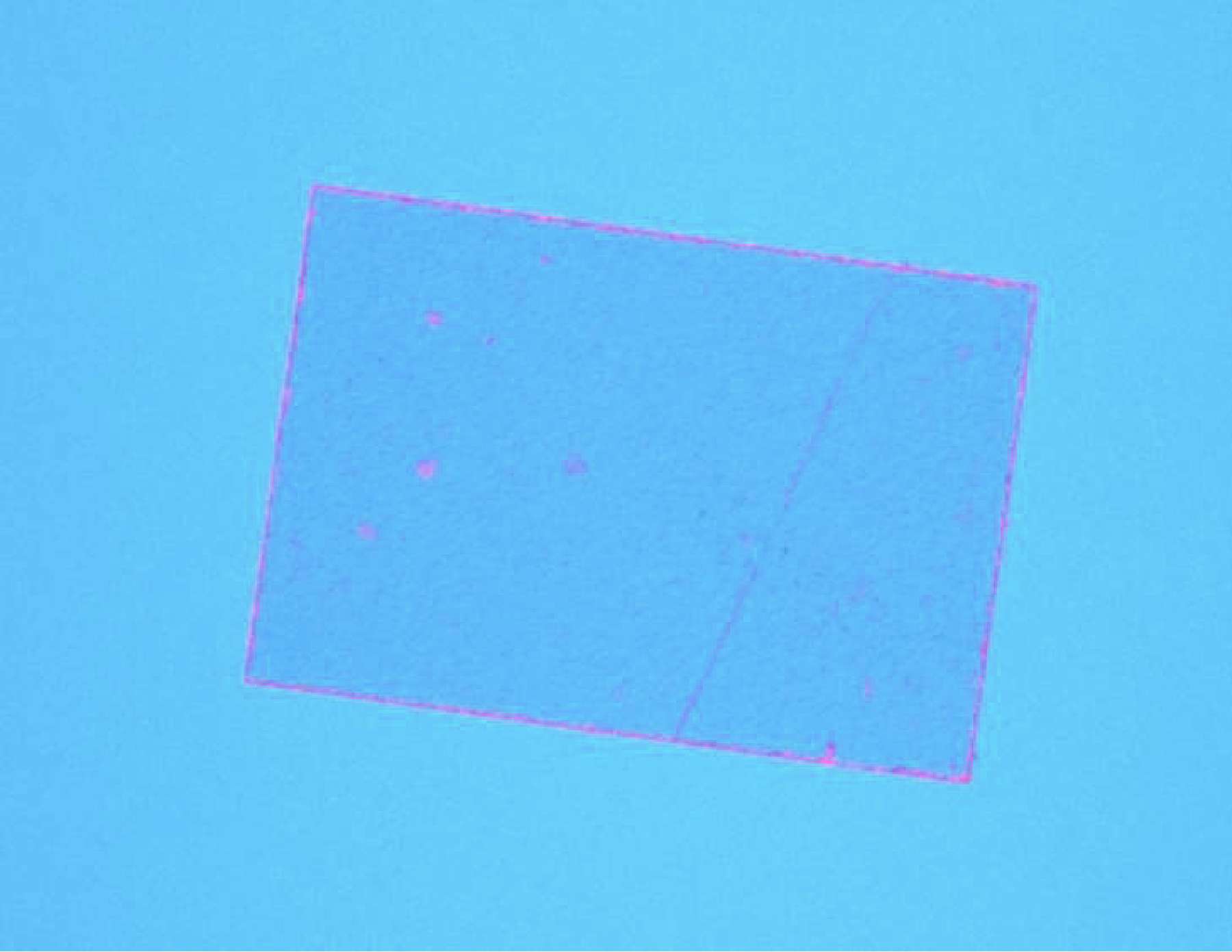
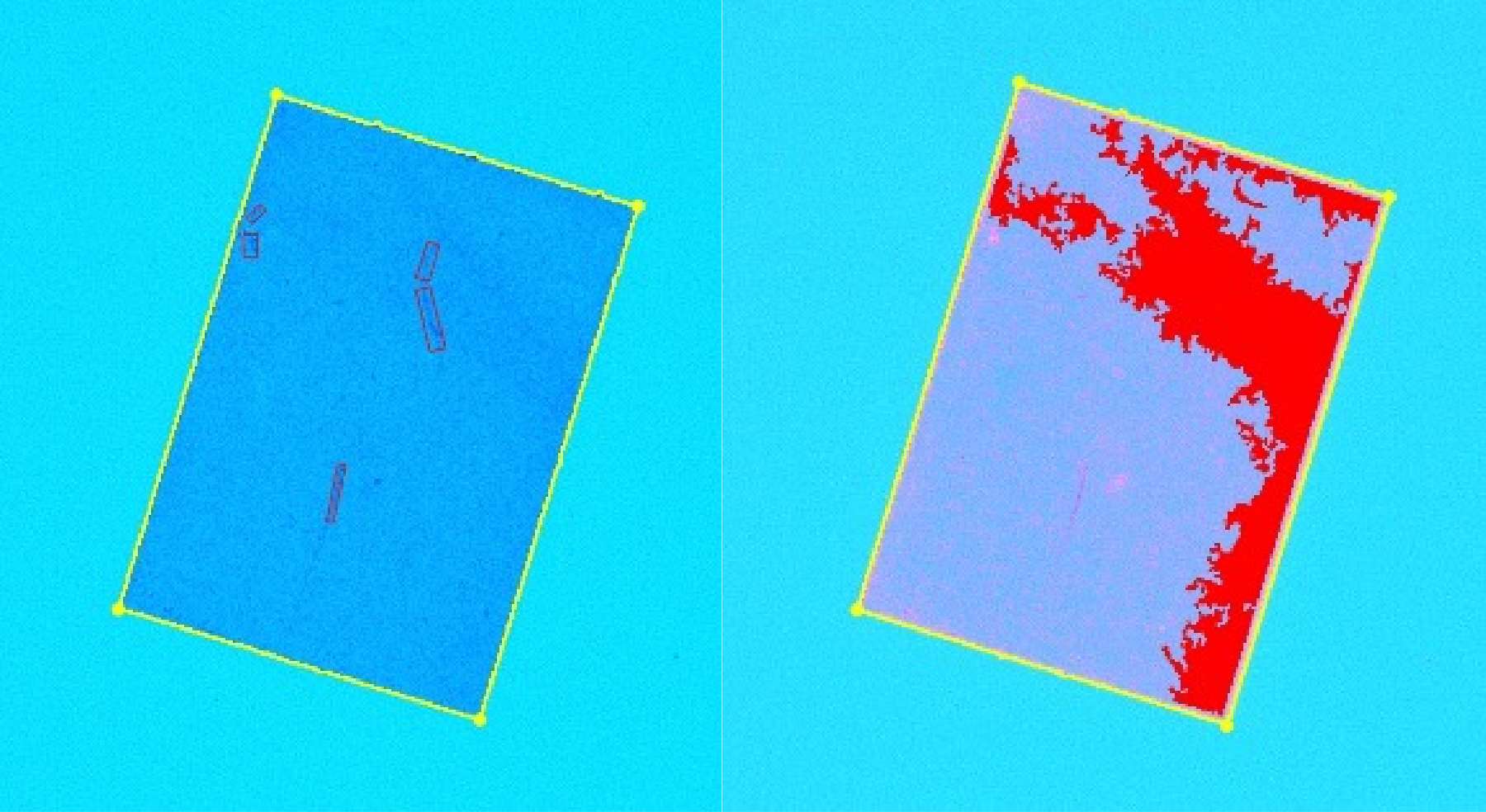